Selected Research
I'm boardly interested in causal inference, sensitivity analysis, optimization, and applications in finance and biology.
|
|
Bounds on the Distribution of a Sum of Two Random Variables: Revisiting a problem of Kolmogorov with application to Individual Treatment Effects.
Zhehao Zhang,
Thomas Richardson
We revisit the following problem, proposed by Kolmogorov: given prescribed marginal distributions F and G for random variables X,Y respectively, characterize the set of compatible distribution functions for the sum Z=X+Y.
Kolmogorov's problem is closely related to inferring possible distributions for individual treatment effects given the marginal distributions of Y1 and Y0; the latter being identified from a randomized experiment.
|
|
Individual treatment effect: distribution bounds, predictive intervals and implications.
Zhehao Zhang,
Thomas Richardson
We study the individual treatment effect (ITE) in causal inference settings and provide partial identification results,
bounds on distribution effects and decision-making discussions. We utilize copulas and Strassen's theorem in our proof. Preprint will be available on ArXiv soon.
|
|
Early Epidemiological Evidence of Public Health Value of WA Notify, a Smartphone-based Exposure Notification Tool: Modeling COVID-19 Cases Averted in Washington State.
Courtney Segal,
Zhehao Zhang,
Bryant T Karras,
Debra Revere,
Gregory Zane,
Janet G Baseman
We assess the impact of
Washington State’s exposure notification tool, WA Notify, in mitigating the spread of COVID-19
during its first four months of implementation.
|
|
A Comparison of Maxillofacial and Head Injuries Following Electric Scooter and Bicycle Accidents.
Brian Bhaskar,
Joshua Alumbaugh,
Zhehao Zhang,
Jasjit Dillon,
Andrea Burke
We compare maxillofacial and head injury location, type and severity related to electric scooters and bicycle accidents and to identify factors contributing to injury severity.
|
|
Segmentation Optimization and Isocenter Selection.
Zhehao Zhang,
Zhixu Tao
,
Matthew R. Walker
,
Mojgan Hodaie
We provide a comprehensive examination of current automatic segmentation techniques on MRI images. We also discuss optimization methods for isocenter selection.
|
|
Inferring Rate Functions for Stochastic Models
of Biological Processes.
Zhehao Zhang,
Jeremy D’Silva
,
Nobuaki Masaki,
Andreas Hilfinger
We used stochastic models to simulate chemical reactions in cells. Derived equations to solve for rates under different models and conducted data simulation.
|
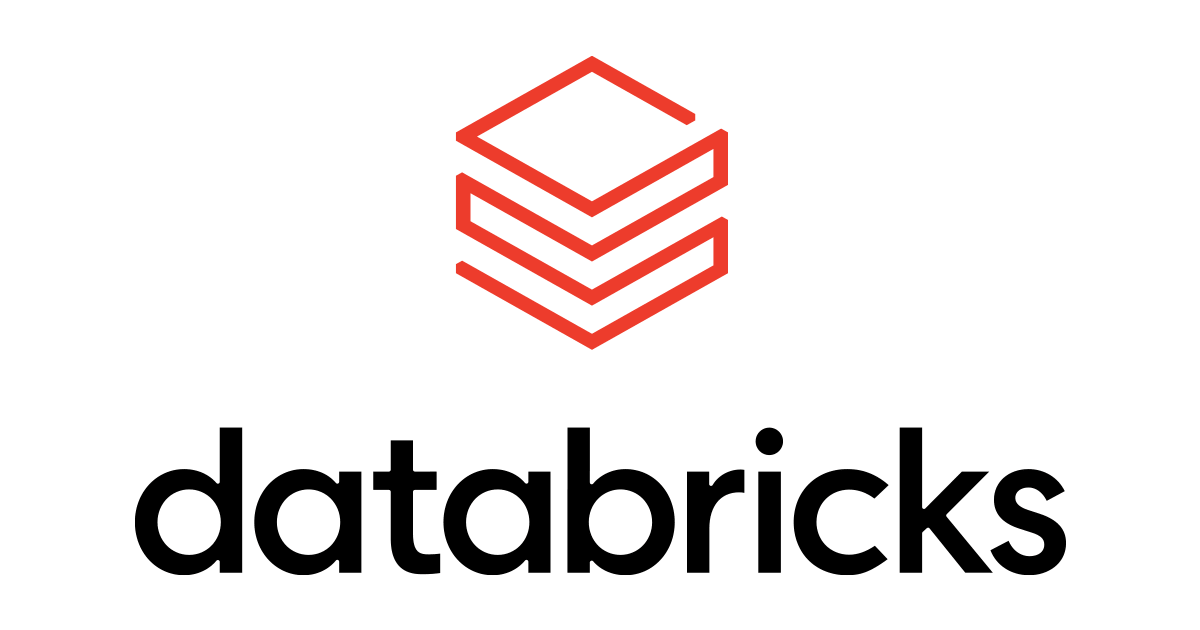 |
Data Science Intern (Summer 2024).
Data Science team at Databricks (work on revenue prediction).
Hosted by Zubair Anwar and Jianda Zhou.
|
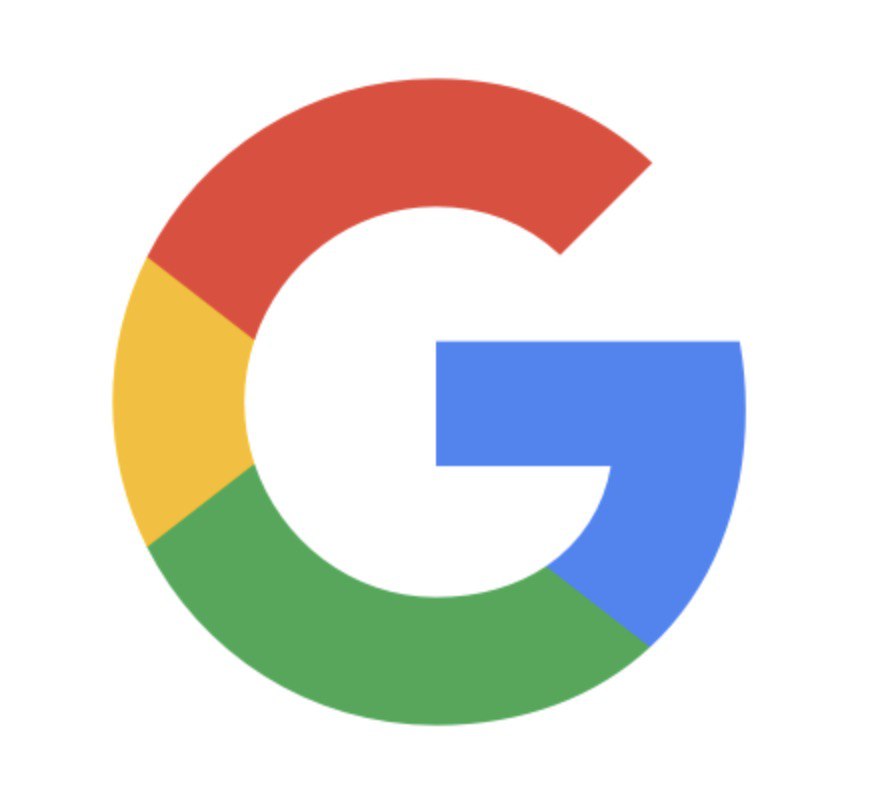 |
PhD Software Engineer Intern (Summer 2022).
Google Cloud Hub Search Team (help launched gmail/chat search chips feature).
Hosted by Sean Ryan and Nick Scoliard.
|
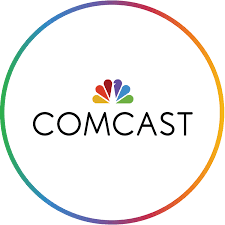 |
Graduate Research Intern (Summer 2021).
Comcast Applied AI team (work on xFinity WiFi optimization).
Hosted by Hongcheng Wang and Yonatan Voizman.
|
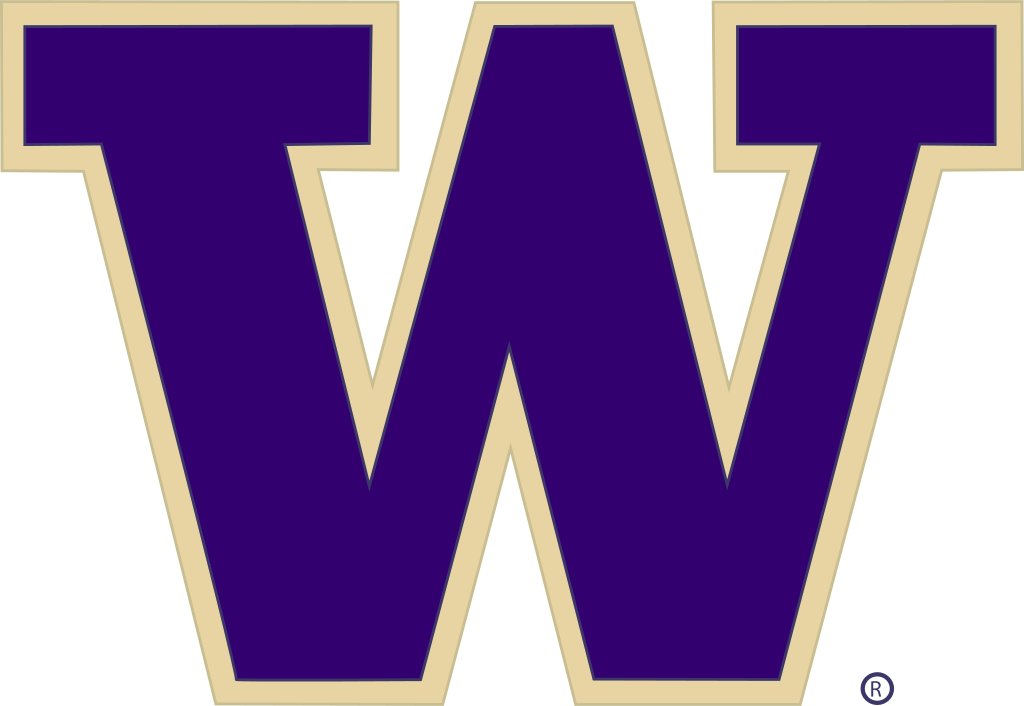 |
Undergraduate Classes:
STAT 311 Elements of Statistical Methods (Fall 2020, Winter 2021)
STAT/MATH 396 A Finite Markov Chains and Monte-Carlo Methods (Spring 2022)
|
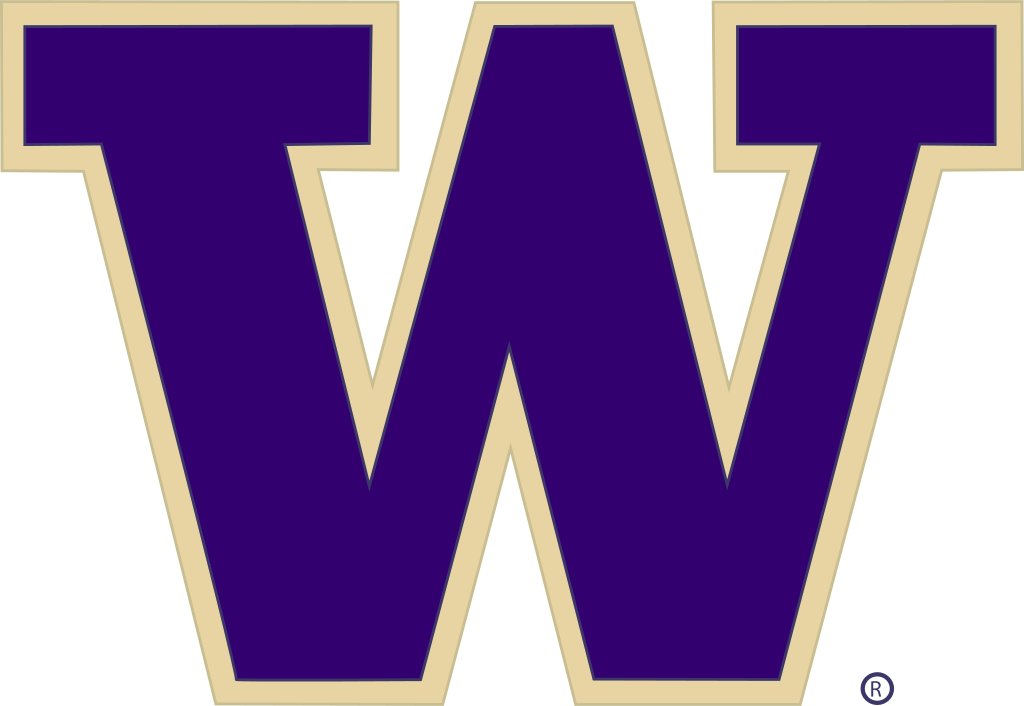 |
Graduate Classes:
STAT 502 Design and Analysis of Experiments (Fall 2022)
STAT 504 Applied Regression (Winter 2022, 2023)
STAT 566 Causal Modeling (Spring 2023)
STAT 570 Advanced Regression Methods for Independent Data (Fall 2023)
STAT 571 Advanced Regression Methods for Dependent Data (Winter 2024)
|
|